In the realm of technology, artificial intelligence stands as a beacon of potential amidst a sea of routine tasks, silently transforming the fabric of daily life by shouldering jobs once held firmly by human hands. You’re living in an era where your interactions with AI automation are as commonplace as they are imperceptible, from the curated newsfeed on your social media accounts to the subtle orchestration of logistics that ensures your packages arrive on time. At its core, AI automation hinges on algorithms that can learn, adapt, and execute tasks with a level of precision and efficiency that’s often beyond human capability. Yet, the intricacies of how these digital minds operate remain a mystery to many. As you navigate through your day, you’re unwittingly standing at the intersection of convenience and complexity, where understanding the cogs of AI automation can unlock a deeper appreciation of the invisible forces shaping your world. Stick with this exploration to unveil the layers that make AI automation not just a technological marvel, but a pivotal element in the evolution of modern society.
Key Takeaways
- Machine learning algorithms are essential for AI automation, allowing systems to learn and make predictions.
- Transparency and accountability are crucial in AI systems, ensuring algorithmic fairness and mitigating biases.
- AI automation has revolutionized various sectors, such as manufacturing, customer service, healthcare, and finance.
- AI technologies aim to augment human capabilities, enhancing productivity and innovation in various industries.
Understanding AI Fundamentals
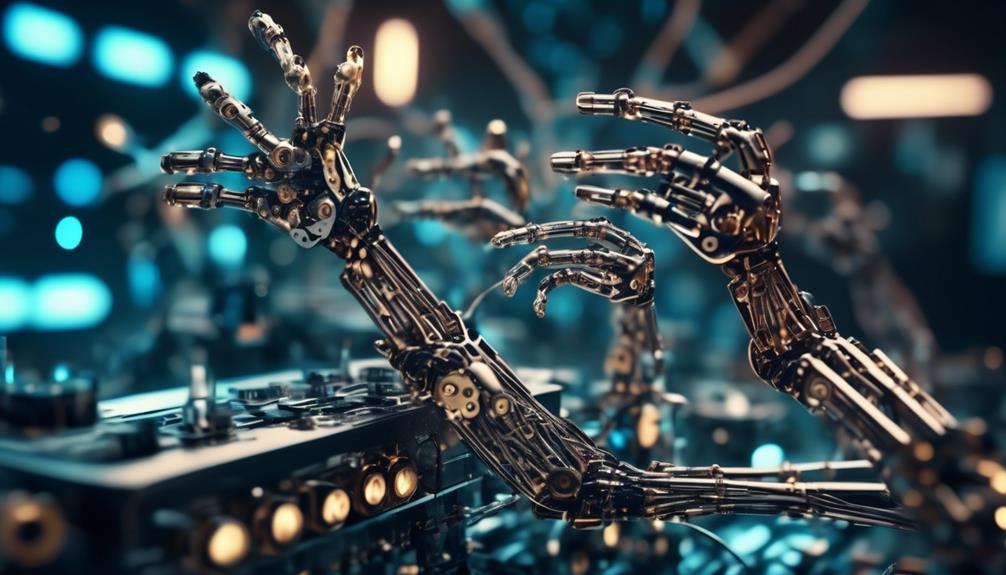
To grasp the mechanics of AI automation, you must first understand its core components, which include machine learning algorithms, data processing techniques, and neural networks. Machine learning algorithms are the backbone of AI, enabling systems to learn from and make predictions on data. You’re dealing with complex statistical models that adjust their parameters based on the input data they receive. This adaptability is crucial for the functionality of AI in diverse applications.
Data processing techniques are equally essential, as they precondition data for optimal algorithm performance. You’re looking at a meticulous process of cleaning, normalizing, and segmenting data to ensure quality and relevance for the task at hand. Without robust data processing, even the most sophisticated algorithms can falter, leading to inaccurate outputs.
Neural networks, inspired by human brain architecture, are pivotal in handling tasks like pattern recognition and decision-making. You’ll find these networks composed of interconnected nodes, mirroring neurons, which process information in layers to extract features and solve complex problems.
Machine ethics come into play when discussing the decision-making aspect of AI automation. You’re responsible for ensuring that the systems you develop adhere to ethical guidelines, which entails transparency in algorithms. Algorithm transparency means you’re making the functioning of AI systems understandable and accountable, which is critical in maintaining trust and mitigating biases in automated decisions.
Key Technologies in Automation
Often, the efficiency and effectiveness of AI automation hinge on the deployment of key technologies such as robotic process automation (RPA), natural language processing (NLP), and cognitive computing. These technologies collectively facilitate the creation of systems that can perform tasks without human intervention, analyze large volumes of data, and make decisions based on complex algorithms.
In particular, RPA plays a crucial role in automating repetitive tasks. It uses bots to emulate the actions a human would take within digital systems, thus streamlining business processes. Meanwhile, NLP enables machines to understand and respond to human language, allowing for more intuitive user interactions. Cognitive computing, on the other hand, mimics human thought processes in a computerized model, enabling systems to solve problems without explicit programming.
Consider these critical technologies:
- Machine Vision: Empowers computers to interpret and process visual data, enhancing quality control in manufacturing.
- Robotic Process Automation: Automates routine tasks, increasing operational efficiency.
- Natural Language Processing: Facilitates human-like understanding of language within applications, improving customer service.
- Cognitive Computing: Implements self-learning algorithms that use data mining and pattern recognition to mimic the human brain.
Learning: AI’s Core Ability
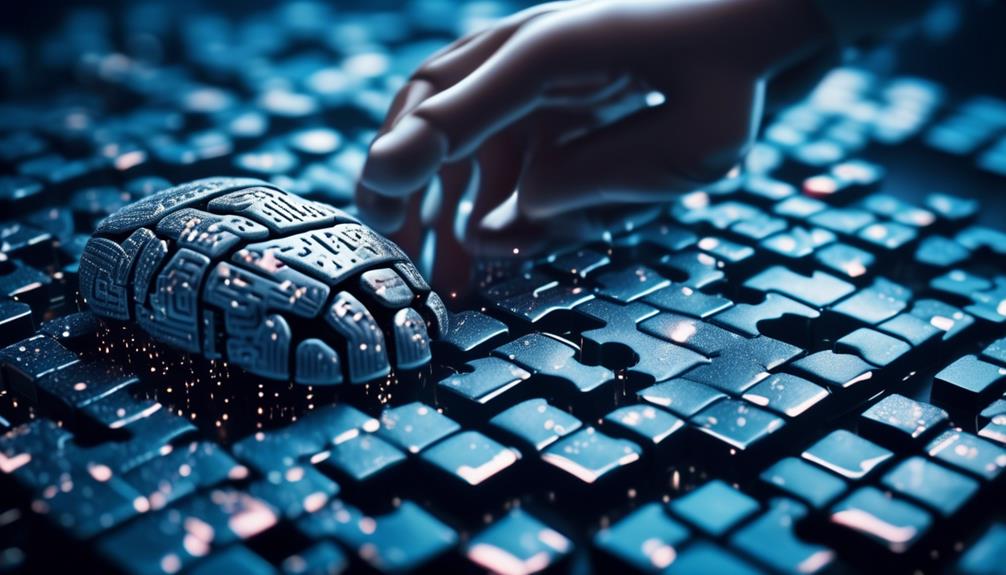
One of AI automation’s most critical capabilities is its ability to learn from data, adapt to new situations, and improve over time without explicit human instruction. This learning is grounded in algorithms that can detect patterns, make decisions, and refine their behavior through a feedback loop known as machine learning. It’s important to understand that this isn’t machine consciousness; AI doesn’t “think” or “understand” in the human sense. Instead, it processes vast amounts of data and applies learned patterns to similar scenarios. AI automation’s ability to learn and improve over time relies heavily on the quality and quantity of data it can access. Effective data collection methods are crucial for providing AI systems with the information they need to make accurate decisions and predictions. As technology continues to advance, the development of more sophisticated data collection methods will be essential to ensure that AI automation continues to evolve and improve in its capabilities.
However, as you delve deeper into the mechanics of AI learning, you encounter the challenge of algorithm bias. Bias can creep into AI systems through the data they’re fed. If the input data reflects historical biases or lacks diversity, the AI’s decisions may be prejudiced, perpetuating existing inequalities. It’s critical to employ rigorous data analysis and algorithm auditing to detect and correct these biases.
Your role in managing AI includes ensuring that the systems are not only efficient but also fair and unbiased. You’re tasked with the continuous analysis of the AI’s performance, adjusting datasets, and algorithm parameters to mitigate bias, all while keeping an eye on the evolving landscape of AI capabilities and ethical considerations.
AI Decision-Making Processes
AI decision-making processes hinge on complex algorithms that analyze data and output choices based on predefined objectives and learned information. As you delve into the intricacies of AI automation, it’s crucial to understand the components that drive these decision-making systems. These algorithms are tailored to parse through vast datasets, identifying patterns and making predictions or decisions accordingly.
However, the efficiency of AI decision-making is not without its challenges. Key issues include:
- Algorithmic bias: Decisions are as good as the data provided. Biases in data can lead to skewed outcomes, affecting the fairness of decisions.
- Ethical considerations: Decision-making models must align with ethical norms, which can be complex when considering diverse cultural and social values.
- Transparency: Understanding the “why” behind decisions is essential, especially in critical applications.
- Adaptability: Algorithms must adjust to new data, ensuring that decisions remain relevant over time.
Algorithmic bias and ethical considerations are particularly poignant. They demand rigorous scrutiny to ensure that automated decisions do not perpetuate existing societal biases or infringe on ethical principles. AI systems must be designed with mechanisms to identify and mitigate bias, and developers must consider the broader implications of their design choices.
Real-World Applications and Impacts

In the landscape of modern technology, AI automation has permeated various sectors, revolutionizing how tasks are performed and decisions are made. This transformation isn’t just innovative—it’s a testament to industry disruption, where traditional processes are overhauled for efficiency and accuracy. You’ve seen it in manufacturing, where robotics equipped with AI perform complex assembly tasks, minimizing human error and maximizing output. In the realm of customer service, chatbots powered by natural language processing handle queries, providing instant support and freeing up human agents for more intricate issues.
This shift isn’t without its challenges, particularly in terms of job evolution. Roles that once relied heavily on repetitive manual input are now transitioning to require oversight of automated systems and data analysis. You’re observing a surge in demand for skills that align with these AI-driven environments—data literacy, software proficiency, and system troubleshooting. It’s not just about displacing jobs, but about creating new opportunities within the evolving technological framework.
The impact extends to healthcare, where AI-driven diagnostics aid in detecting diseases with higher precision, and to finance, where algorithmic trading influences market dynamics. In every instance, the thrust of AI automation is towards augmenting human capabilities, not replacing them, thereby catalyzing an era of enhanced productivity and innovation.
Conclusion
In conclusion, you’ve seen how AI automation hinges on complex algorithms and machine learning. It’s the AI’s ability to learn and adapt that underpins its decision-making, streamlining operations across various industries. By analyzing vast datasets, AI enhances efficiency and accuracy, leading to significant real-world impacts. As you continue to interact with these technologies, it’s crucial to understand their technical nuances, ensuring you can navigate and leverage the automated landscape effectively and responsibly.
About the Author: Thad Paschall is a renowned entrepreneur, CEO, and digital marketing expert with over 30 years of experience in the security industry. As the Founder and CEO of AI Security Edge, an AI-driven digital marketing agency, and the former Founder and CEO of Protect America, a leading home security provider, Thad has revolutionized the industry with his innovative strategies and visionary leadership. With his expertise in AI, automation, and revenue acceleration, Thad is widely recognized as one of the most sought-after experts in the security industry.