To maximize AI’s potential, treat it like an enthusiastic intern. Start by setting clear, measurable expectations with defined goals. Provide structured training using real-world scenarios and regular skill assessments. Conduct performance reviews based on data-driven metrics like precision and recall. Assign tasks that align with AI’s strengths in data analysis and automation. Foster innovation by integrating AI into brainstorming sessions. Implement continuous monitoring with feedback loops and clear benchmarks. Build a collaborative environment that seamlessly integrates AI and human workflows, and celebrate achievements to boost morale. Continue to refine your approach by learning more about each step in detail.
Key Takeaways
- Establish clear, measurable goals and expectations for AI tasks and outputs.
- Provide structured training and regular assessments to track AI progress.
- Conduct regular performance reviews using specific metrics like precision and recall.
- Assign tasks that align with AI’s strengths in data analysis and automation.
- Foster a collaborative environment integrating AI seamlessly with human workflows.
Setting Clear Expectations
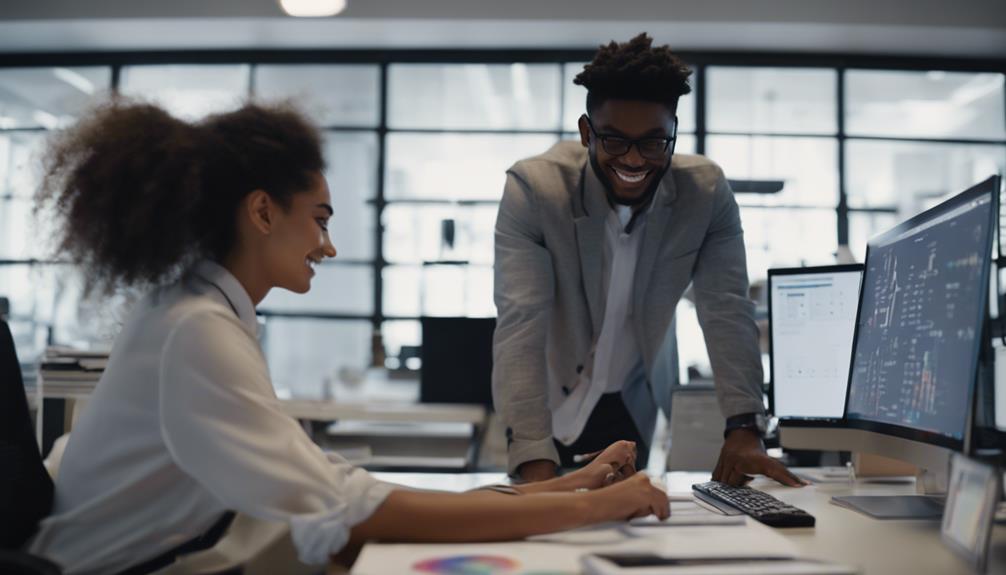
To ensure your AI performs at its best, start by setting measurable and specific expectations for its tasks and outputs. Establishing clear goals is vital for guiding the AI’s development and ensuring it operates within desired parameters. Begin by defining precise objectives. Use data-driven metrics to set performance benchmarks, such as accuracy rates, response times, or error margins. This approach provides a concrete framework for evaluating the AI’s effectiveness and progress.
Communication clarity is essential in this process. Clearly articulate the tasks the AI needs to perform and the expected outcomes. Avoid vague or ambiguous instructions; instead, use specific language that leaves no room for misinterpretation. For example, if the AI is tasked with data analysis, specify the types of data, the analysis methods to be used, and the format of the desired results.
Incorporate feedback loops to regularly review performance against the set goals. This analytical approach helps in identifying areas for improvement and adapting expectations as the AI evolves. By focusing on goal setting and maintaining communication clarity, you create a structured environment where your AI can thrive and deliver excellent results.
Providing Proper Training
To guarantee your AI system performs at its best, it’s essential to implement structured learning modules tailored to its specific tasks. Regular skill assessments will help you monitor progress and identify areas needing improvement, ensuring continuous development. By adopting a data-driven training approach, you can fine-tune the AI’s capabilities efficiently.
Structured Learning Modules
Consistently delivering effective AI performance requires implementing structured learning modules that provide thorough and systematic training. By crafting a personalized curriculum tailored to your AI’s specific needs, you can guarantee a more precise and efficient learning experience. Structured learning modules should outline clear learning milestones to track progress and identify gaps.
For a successful implementation, consider these key elements:
- Data-Driven Content: Use relevant datasets that reflect real-world scenarios your AI will encounter.
- Incremental Complexity: Start with simple tasks and gradually introduce more complex challenges to build competency.
- Feedback Loops: Incorporate mechanisms for immediate feedback to correct errors and reinforce accurate performance.
- Regular Updates: Continuously update the curriculum to reflect new advancements and emerging data trends.
A technical and analytical approach ensures that each aspect of the training module is aligned with your AI’s operational goals. By setting distinct learning milestones, you can systematically evaluate the progress, making adjustments as necessary to maintain the trajectory towards peak performance. This method not only maximizes the efficiency of your AI training but also ensures that your AI remains adaptable and responsive to changing requirements.
Regular Skill Assessments
Regular skill assessments are crucial for guaranteeing your AI remains effective and continually improves its performance. By conducting regular competency evaluations, you can identify areas where the AI excels and where it needs further training. This data-driven approach allows you to set accurate proficiency benchmarks and track progress over time.
To begin, establish clear metrics for evaluating your AI’s performance. These could include accuracy, response time, and error rates. Regularly scheduled competency evaluations will help you gather quantitative data, which is essential for analyzing your AI’s strengths and weaknesses. Use this data to adjust training modules and focus on areas needing improvement.
Next, compare your AI’s performance against industry standards and internal proficiency benchmarks. This benchmarking process will offer valuable insights into how well your AI stacks up against its peers and its previous iterations. Continuous comparison ensures that your AI is not just meeting but exceeding expectations.
Regular Performance Reviews
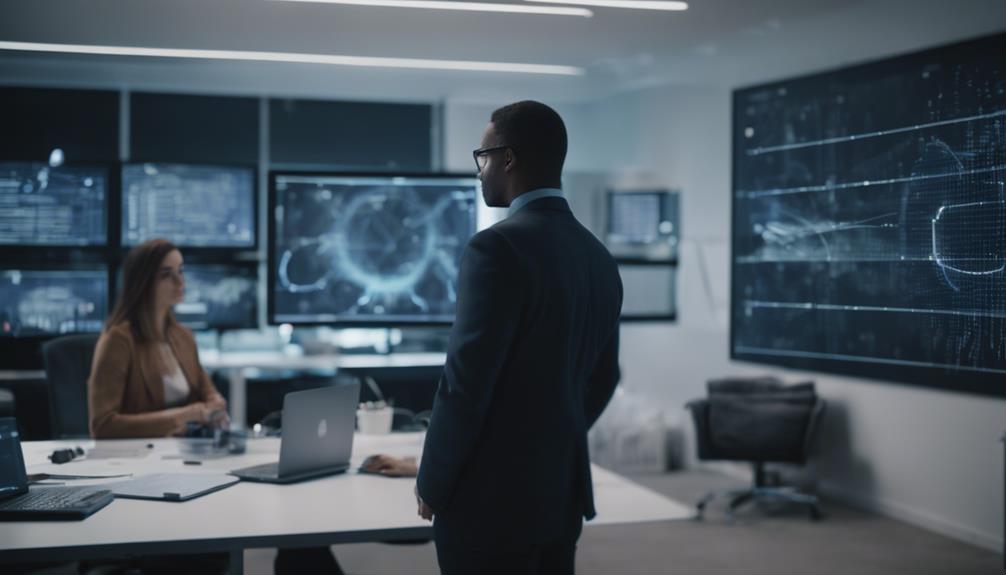
You should conduct regular performance reviews to establish clear expectations for your AI system and encourage continuous improvement. Use data analytics to assess its accuracy, efficiency, and areas needing refinement. This structured approach guarantees that your AI aligns with your evolving business goals.
Setting Clear Expectations
Establishing clear expectations through regular performance reviews guarantees AI systems align with your organizational goals and adapt to evolving requirements. By setting precise benchmarks and employing data-driven assessments, you ensure that your AI consistently delivers value. Effective communication channels are essential for this process, enabling you to relay objectives and receive feedback seamlessly.
Regular performance reviews should consider the following:
- Metrics and KPIs: Define specific performance indicators to measure the AI’s effectiveness.
- Goal Alignment: Ensure that the AI’s tasks and outcomes are in sync with your strategic goals.
- Feedback Loops: Implement structured feedback mechanisms to address issues promptly.
- Adaptation Strategies: Develop plans for the AI to adapt to new data or changing requirements.
Utilize an analytical approach to monitor the AI’s outputs and adjust its parameters as needed. Regular reviews not only highlight areas for improvement but also reinforce successful strategies. Use quantitative data to back up your assessments, ensuring objectivity and precision. This structured review process fosters a continuous cycle of evaluation and optimization, keeping your AI aligned with your evolving goals. By embedding these practices into your workflow, you can maximize the efficiency and effectiveness of your AI systems.
Encouraging Continuous Improvement
Continuous improvement in AI performance hinges on systematically analyzing data and implementing iterative enhancements. You need to adopt a growth mindset to guarantee your AI model evolves efficiently over time. Regular performance reviews are essential for identifying areas where your AI can improve. By providing iterative feedback, you can fine-tune algorithms and enhance overall accuracy.
To facilitate these reviews, create a structured approach:
Review Aspect | Metrics to Analyze |
---|---|
Accuracy | Precision, Recall, F1 Score |
Efficiency | Processing Time, Resource Usage |
User Interaction | User Satisfaction Score, Error Reports |
Adaptability | Error Reduction Rate, Learning Speed |
Scalability | Load Handling, Response Time |
In your performance reviews, focus on data-driven insights. Track metrics like precision, recall, and F1 score to gauge accuracy. Evaluate processing time and resource usage for efficiency insights. Examine user satisfaction scores and error reports to improve user interaction. Assess error reduction rate and learning speed to measure adaptability. Lastly, analyze load handling and response time to ensure scalability.
Iterative feedback is key. Regularly update your AI model based on these metrics, fostering a culture of continuous improvement. By systematically analyzing performance and embracing a growth mindset, you’ll guarantee your AI remains robust and effective.
Assigning Appropriate Tasks
When assigning tasks to an AI, make sure they align with its strengths in data analysis, pattern recognition, and automation to maximize efficiency and accuracy. The key to effective task delegation is understanding the AI’s capabilities and limitations, and then matching those with the right responsibilities. This guarantees that the AI can perform at its best, providing you with reliable and timely outputs.
Consider these types of tasks for your AI:
- Data Analysis: Use AI to sift through large datasets, identify trends, and generate actionable insights.
- Pattern Recognition: Assign tasks that require identifying patterns in data, such as fraud detection or predictive maintenance.
- Automation: Implement AI in repetitive tasks like data entry, report generation, or customer service interactions.
- Optimization: Delegate tasks that involve optimizing processes and resources, such as supply chain management or scheduling.
Encouraging Innovation
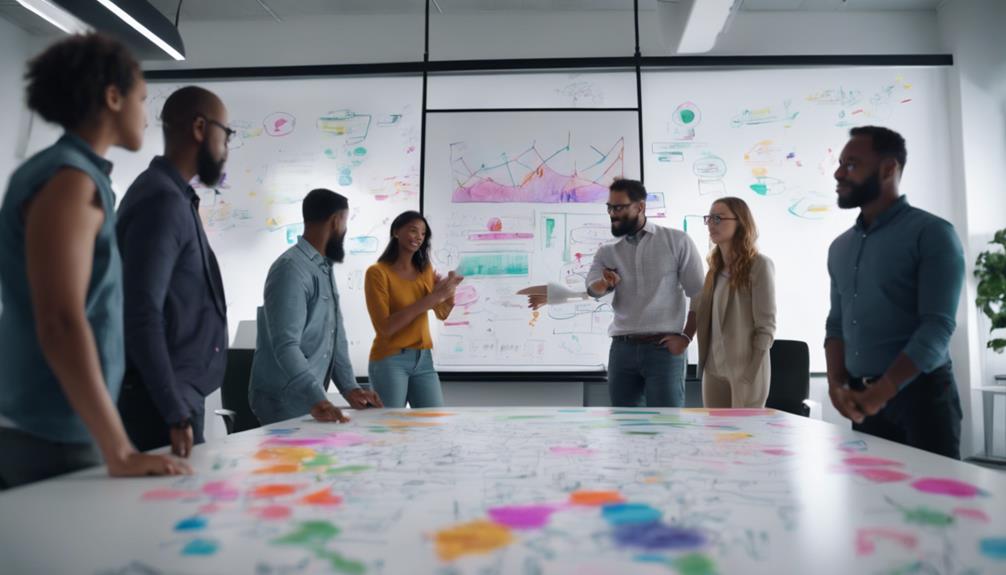
To foster innovation with AI, leverage its ability to analyze extensive datasets and uncover hidden opportunities that humans might overlook. Begin by integrating AI into cross-departmental brainstorming sessions. AI’s data-driven insights can provide fresh perspectives that fuel creative freedom and spark novel solutions. For instance, employ machine learning algorithms to identify patterns in customer behavior across marketing, sales, and product development departments. Consider partnering with an AI digital marketing agency to further enhance your innovation efforts. These agencies specialize in using AI and data analytics to optimize marketing strategies and uncover new ways to engage with customers. By tapping into their expertise, you can gain valuable insights and ideas for leveraging AI to drive innovation in your organization.
Encourage your teams to use AI-generated analytics to challenge conventional thinking. AI can quickly analyze large volumes of data, revealing correlations and trends that aren’t immediately apparent. By presenting these insights during brainstorming sessions, you enable team members to think outside traditional boundaries, fostering a culture of innovation.
Ensure that your AI tools have access to expansive datasets spanning multiple departments. This holistic approach allows AI to draw connections across different functional areas, enriching the brainstorming process. Additionally, give your AI the creative freedom to suggest unconventional solutions. Sometimes, the most groundbreaking ideas come from unexpected correlations and insights.
Monitoring and Feedback
As your team harnesses AI to drive innovation, it’s essential to implement robust monitoring and feedback mechanisms to assess its effectiveness and guarantee alignment with organizational goals. Real-time oversight is vital for identifying any deviations or inefficiencies promptly. By continuously monitoring AI outputs, you can make sure the system remains on track.
To achieve this, consider the following strategies:
- Implement Continuous Monitoring: Use dashboards and real-time analytics to track AI performance metrics, allowing for immediate intervention when necessary.
- Establish Clear Benchmarks: Define specific KPIs that align with your business objectives to measure AI’s success accurately.
- Regular Audits: Conduct periodic reviews of AI processes and outcomes to identify areas for improvement.
- Feedback Loops: Develop a system for providing constructive criticism to the AI, enabling it to learn and adapt based on real-world performance.
A data-driven, analytical approach to monitoring will help you pinpoint areas where the AI excels and where it needs improvement. Constructive criticism, when integrated into feedback loops, will foster continuous learning and adaptation. By maintaining real-time oversight and leveraging structured feedback, you make sure that your AI system not only meets but exceeds organizational expectations.
Building a Collaborative Environment
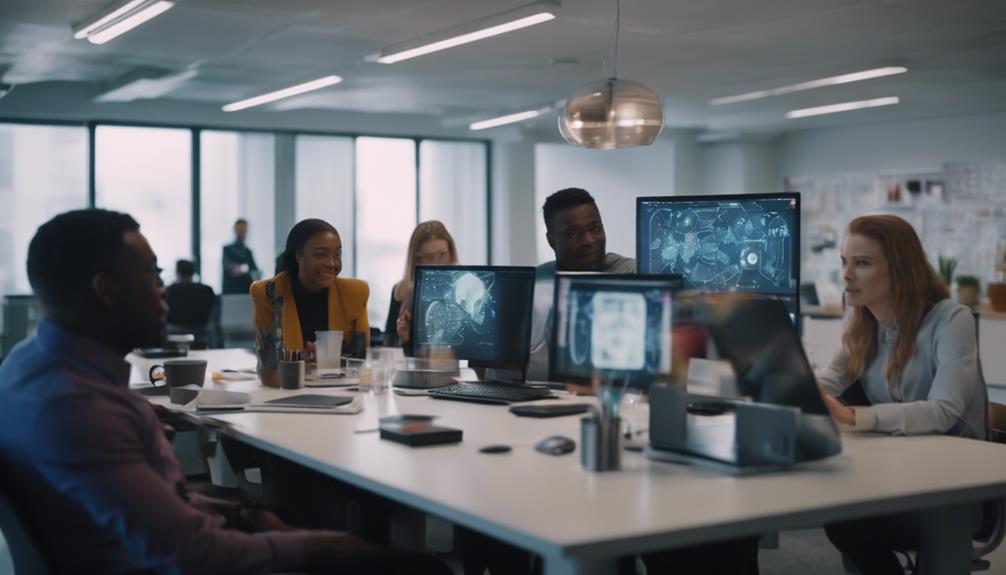
Fostering a collaborative environment requires integrating AI seamlessly with human workflows, ensuring that both entities complement each other’s strengths. You’ll need to focus on creating a team culture that values both human expertise and AI capabilities. Start by establishing clear roles for AI and human team members. For instance, let AI handle data analysis and repetitive tasks while humans focus on strategic decision-making and creative problem-solving. This delineation leverages the strengths of both parties.
Open communication is critical. Encourage team members to share insights and observations about AI’s performance. Use data-driven feedback to refine AI models and adjust workflows accordingly. Regular meetings and collaborative platforms can facilitate this exchange of information.
Quantitative metrics should be employed to assess how well AI integrates into the team. Track key performance indicators (KPIs) like task completion times, error rates, and user satisfaction scores. This analytical approach will provide a clear picture of AI’s impact on productivity and team dynamics.
Celebrating Achievements
Consistently celebrating achievements guarantees that both human and AI contributions are recognized and valued, fostering a culture of mutual respect and continuous improvement. This approach isn’t just about boosting morale; it’s also rooted in data-driven strategies that enhance productivity and innovation. Recognizing milestones and sharing accomplishments leads to measurable benefits, such as increased engagement and optimized performance metrics.
Here are four key benefits of celebrating achievements:
- Increased Motivation: Regular acknowledgment of both human and AI contributions boosts team morale and drives further innovation.
- Enhanced Collaboration: Sharing accomplishments fosters a sense of unity and encourages collaborative problem-solving.
- Data-Driven Insights: Tracking milestones allows you to analyze performance trends and refine strategies for future projects.
- Continuous Improvement: Recognizing achievements helps identify best practices and areas for enhancement, promoting ongoing development.
When you consistently celebrate milestones, you create a feedback loop that benefits both human employees and AI systems. For instance, AI algorithms can be fine-tuned based on past successes, while human team members feel more connected to the overall mission. By leveraging data analytics to pinpoint key achievements, you make sure that recognition is both meaningful and impactful. This balanced approach guarantees that all contributions are valued, leading to a more robust and innovative team dynamic.
Frequently Asked Questions
How Can AI Handle Sensitive or Confidential Information?
To guarantee AI handles sensitive or confidential information securely, we use data encryption to protect the data and implement strict access controls to limit who can view or modify the information. This approach guarantees robust data security.
What Are the Ethical Considerations When Using AI in the Workplace?
You must implement transparency policies and secure employee consent when using AI in the workplace. These steps guarantee ethical practices, protect privacy, and build trust, minimizing risks associated with data misuse and algorithmic biases.
How Do You Address AI Bias in Decision-Making Processes?
To address AI bias in decision-making processes, you should focus on bias mitigation by ensuring your training data is diverse and representative. Regularly audit and refine algorithms using data-driven methods to identify and correct any biases.
What Are the Potential Risks of Over-Relying on Ai?
Over-relying on AI poses risks like job displacement and creativity limitations. You’ll find that excessive dependence can stifle innovation and reduce human oversight, leading to potentially biased and less holistic decision-making processes.
How Do You Ensure AI Compliance with Industry Regulations?
To guarantee AI compliance with industry regulations, you must align your training data with the evolving regulatory landscape. Continuously monitor changes, update your models accordingly, and implement robust auditing mechanisms to maintain compliance and data integrity.
About the Author: Thad Paschall is a renowned entrepreneur, CEO, and digital marketing expert with over 30 years of experience in the security industry. As the Founder and CEO of AI Security Edge, an AI-driven digital marketing agency, and the former Founder and CEO of Protect America, a leading home security provider, Thad has revolutionized the industry with his innovative strategies and visionary leadership. With his AI, automation, and revenue acceleration expertise, Thad is widely recognized as one of the most sought-after experts in the security industry